2019年3月27日下午3点,应我中心邀请,浙江大学CAD&C国家重点实验室研究员许威威教授和美国新墨西哥大学阿尔布克尔克电气与计算机工程系杨垠副教授来访软件学院做学术讲座。讲座由姚俊峰老师主持,吸引了多名外校学者、本校多位副教授和二十多名博士生、硕士生及本科生参加了讲座,现场气氛热烈!
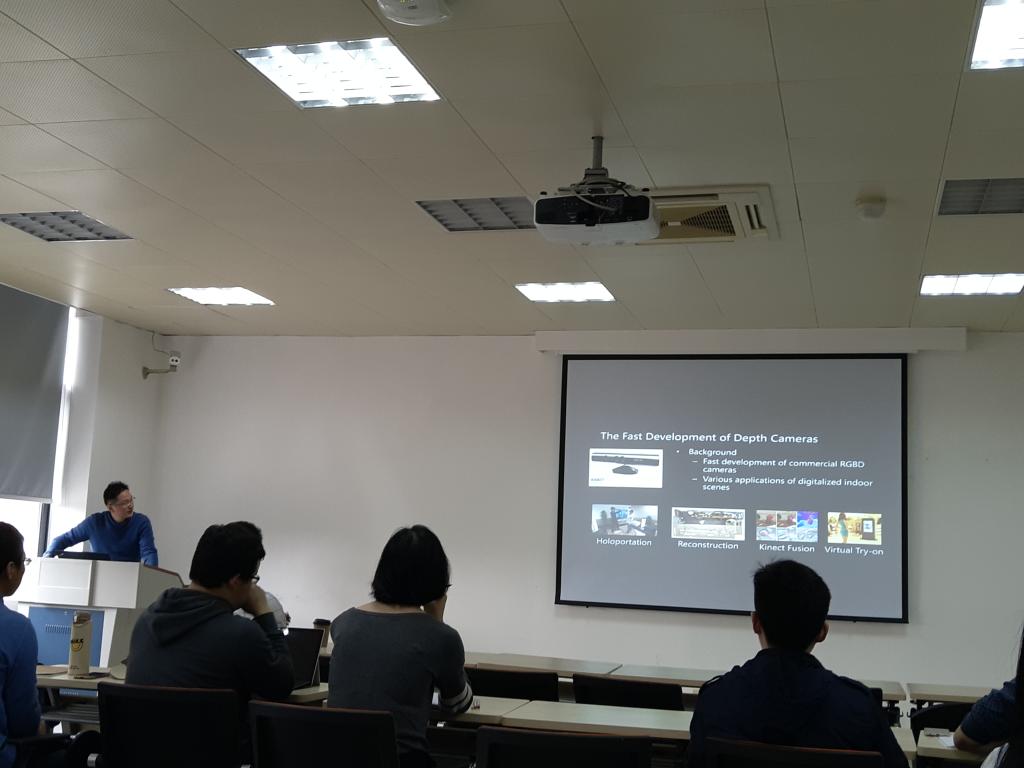
讲者简介:
许威威教授,现任浙江大学CAD&C国家重点实验室研究员,曾任日本立命馆大学博士后,微软亚洲研究院网络图形组研究员, 杭州师范大学浙江省钱江学者特聘教授。主要研究兴趣为三维重建、物理仿真、3D打印和虚拟现实,发表高水平论文近70余篇,其中顶级期刊ACM Transactions on Graphics论文17篇,研究成果在三维扫描仪和人体三维重建等企业产品中得到应用。2014年获国家自然科学基金优秀青年基金资助。
Dr. Yin Yang is currently an Associate Professor with the department of Electrical and Computer Engineering at the University of New Mexico, Albuquerque. Yin received Ph.D. degree of Computer Science from The University of Texas, Dallas in 2013 (he was the awardee of David Daniel Fellowship Prize). He was a Research/Teaching Assistant at UT Dallas as well as UT Southwestern Medical Center. His research mainly focuses on real-time physics-based computer graphics, game, animation and visualization with a strong emphasis on inter- disciplinary research with bio/medical science. He was a Research Intern in Microsoft Research Asia in 2012. Dr. Yang received NSF CAREER award in 2019. Dr. Yang has published over 50 conference/journal articles in areas of computer graphics, animation, computer aided design, and medical imaging. He serves as the TPC member for many international conferences and the reviewer for almost all the top journals/conferences in computer graphics and animation.
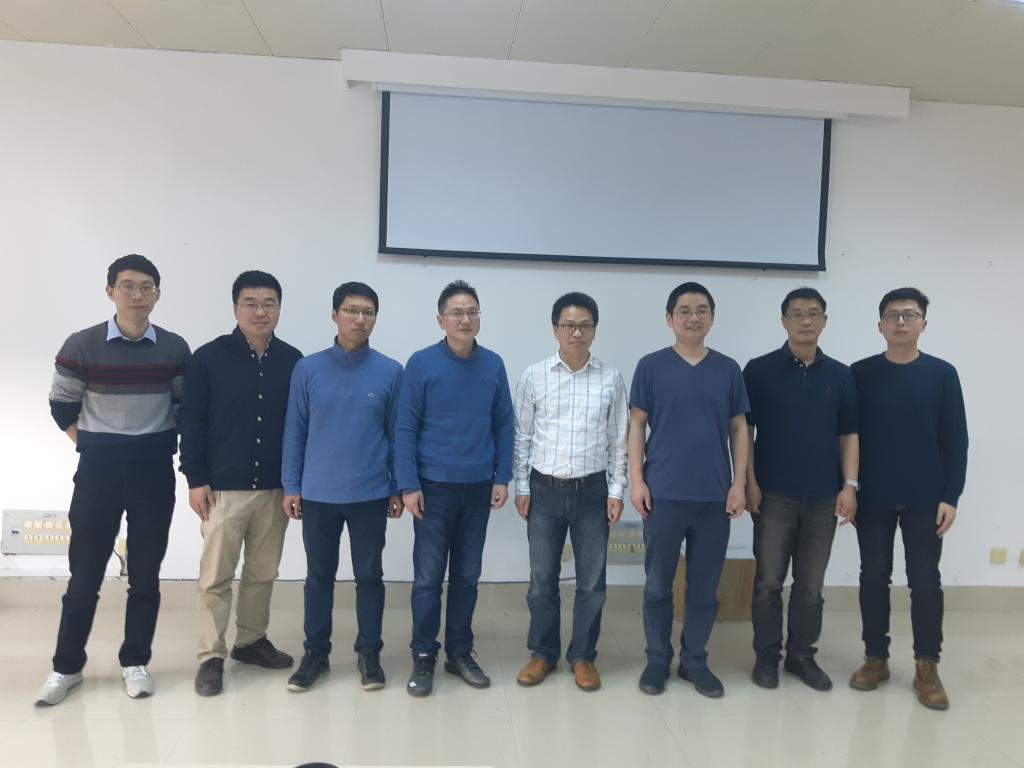
许威威教授主要为大家讲授了,深度相机可直接获取场景三维信息,是兼具便携性和实时性的三维传感器,在人体识别和三维重建中都得到了广泛应用。本报告聚焦于三维重建中深度数据处理的关键技术问题,报告如何结合GPU众核技术对获取的深度数据进行实时处理,包括深度数据噪声去除、实时非刚性全局注册,得到高质量的三维重建结果。针对获取场景数据中存在大量冗余信息的特点,介绍如何分析和提取场景中的特征,进行语义级别的场景重建。
杨垠教授的报告内容主要为:Using the digital computer to simulate dynamic behavior of solid objects is an open problem in many scientific and engineering research: in computer animation, it provides realistic effects of soft characters; in surgical simulation, it delivers vivid visual experiences to the trainee; in digital fabrication, it couples geometry and structural analysis. However, simulating elastic solids with hundred thousands or even millions of degrees of freedom is challenging and computational intensive because one needs to solve a large number of mutually coupled unknowns. In this talk, I will share with some of our recent efforts dealing with this notorious computing challenge: 1) using reduced model, 2) domain decomposition, 3) GPU-based nonlinear solvers and 4) Neural Network and data driven. I will also show you how those algorithms can be integrated into various applications to push forward the boundary of future computing.